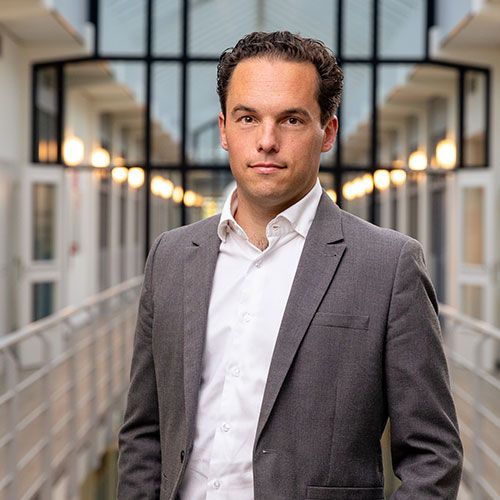
Generate near-infinite permutations of complex, domain-specific, 3D environments
Control systems can vary from simple linear single input single output (SISO) systems to complex non-linear multi-input multi-output (MIMO) systems. While simple systems can easily be controlled by the application of PID controllers, nonlinear MIMO systems are much more complicated.
Traditionally, a lot of effort is put into decoupling inputs and linearizing these nonlinear MIMO systems to make a well-functioning control system. However, reinforcement learning offers a different approach where the algorithm learns the optimal behavior by itself. The challenge is to get high performance in terms of stability and or reaction speed in these non-linear MIMO control systems.
We can use reinforcement learning (RL) techniques to optimize your machine’s performance in two ways. First, use RL to model the entire nonlinear MIMO control system when the system is too complex and non-linearizable. Secondly, use RL to tune settings in the traditional control system to squeeze out the last bit of performance.
Generate near-infinite permutations of complex, domain-specific, 3D environments
Increase functionality by actionable knowledge extraction
More robust machine vision applications
Optimize machine operation quality