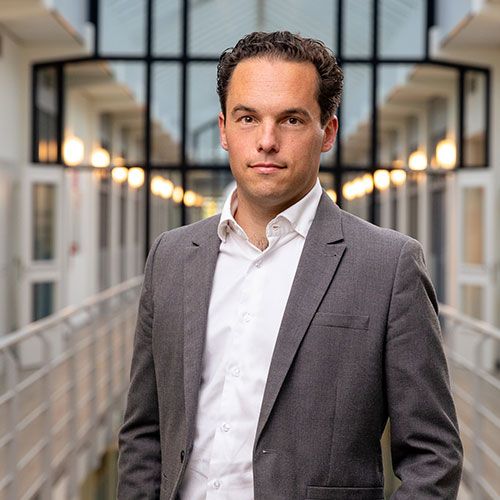
Generate near-infinite permutations of complex, domain-specific, 3D environments
In many engineering applications, a lot of sensor data is being recorded offering a significant potential to obtain useful information and create additional functionalities. We help you unlock the power of sensor data with actionable knowledge extraction.
The term ‘actionable knowledge’ refers to the extraction of information, such as patterns or anomalies, from data that can be used to initiate follow-up actions. For example, identifying anomalous breathing patterns from sensor data (pressures, flows) in a respiratory device to create a warning system for the physician.
In many applications, the available sensor data is however very noisy due to high variability in the use environment or poor sensor quality. Advanced statistical- and machine-learning techniques enable us to extract actionable knowledge in these situations. With the application and integration/embedding of these smart algorithms in your system we can create new functionalities. For example, simultaneously detecting the respiratory effort and heartbeat signal from a single surface electromyography sensor.
Generate near-infinite permutations of complex, domain-specific, 3D environments
More robust machine vision applications
Optimize machine operation quality
Optime control and machine performance