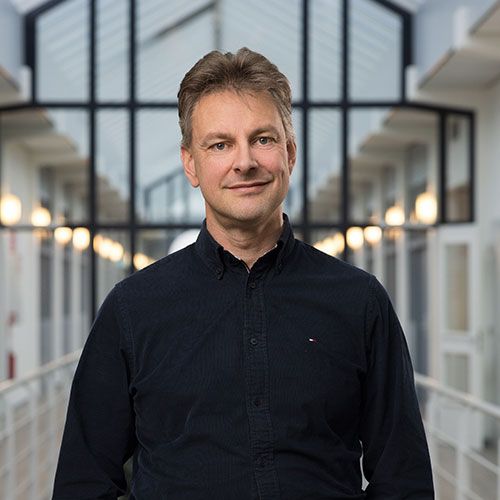
queueing theory and system architecture.
The production line consists of three workstations and a robot to do the logistics: getting the product from one station to the other. Typically, the product needs a few iterations through the line before it’s finished and can be shipped to the customer. The problem was that the products did not flow equally through the different workstations (bottleneck), creating a traffic jam.
With basic queueing theory based on already available logging data of the current production system, we have shown that a different architecture would increase throughput of the system with 50%. Using this data-driven solution, without making significant changes to the physical production line; the main bottleneck was removed because capacity was better balanced and the impact on throughput quantified with recent production data.
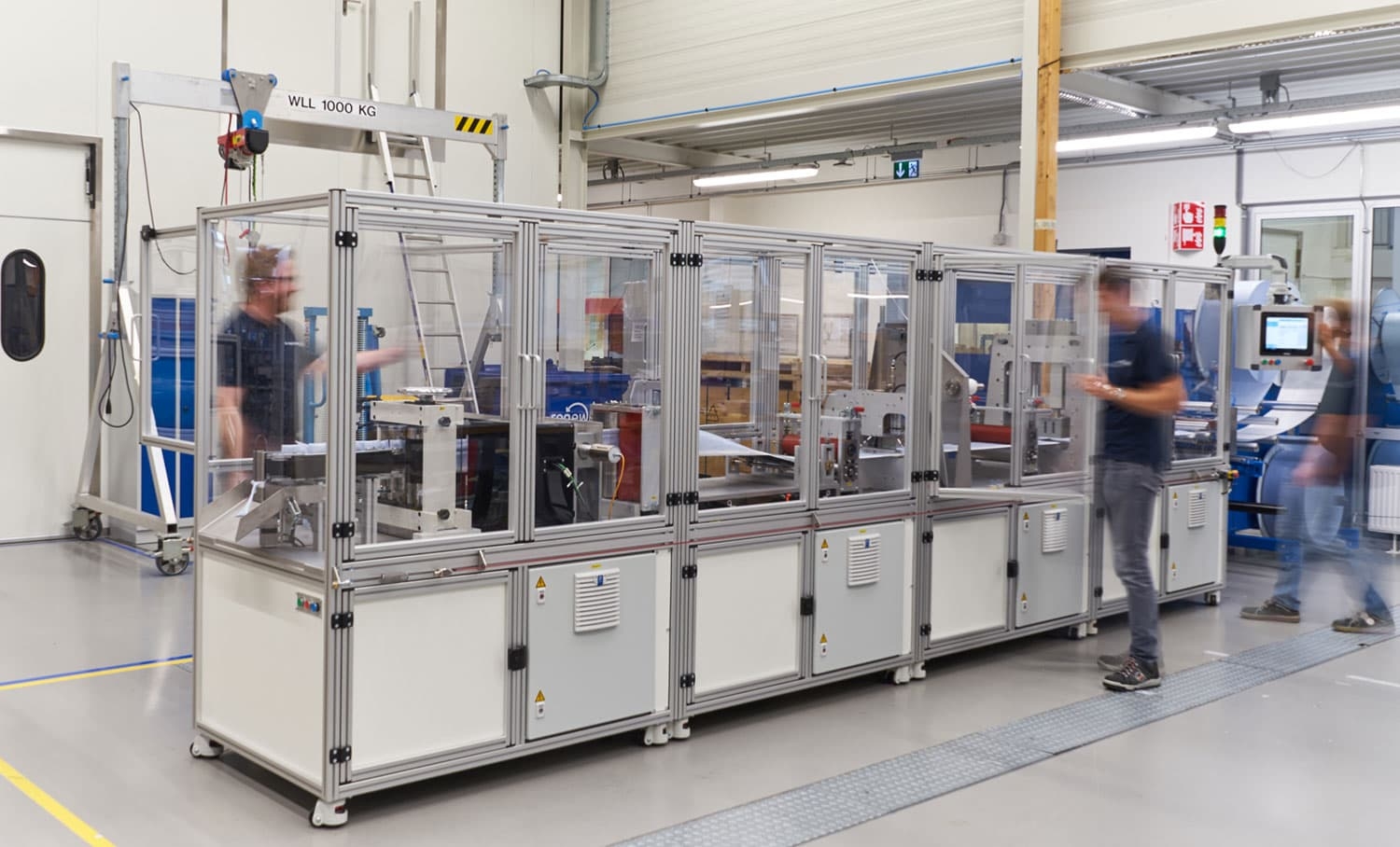
classical versus Deep Learning APC.
Because there was a lot of production data available, we also investigated the added value of Deep Learning compared to classical methods of process learning in this project. It turned out that Deep Learning could have (limited) added value, but not enough worth the effort.
However, we did show that with a classical Advanced Process Control algorithm, learning from past production data also had the potential to increase throughput by another 50%. And again, the data necessary was already available.
"optimizing production process with algorithms based on logging data has huge potential."
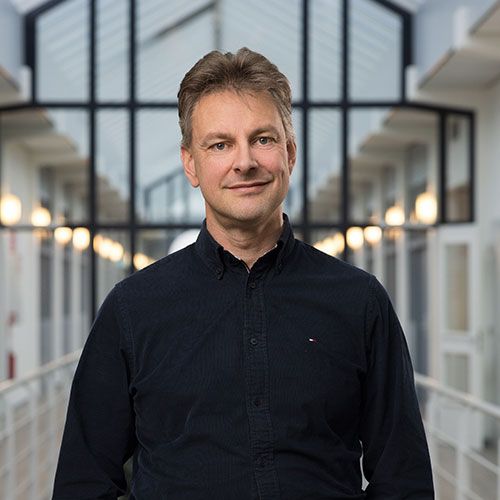